Network-Based Cycling Planning
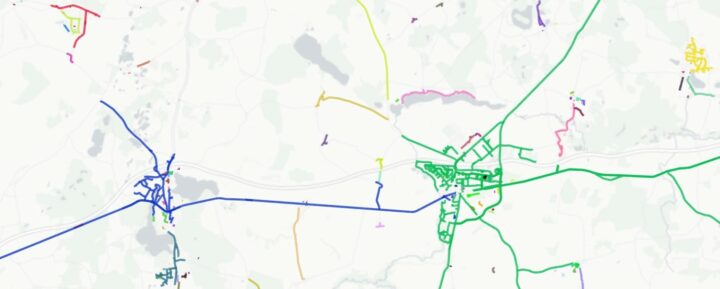
Cohesive cycling networks that balance geographic coverage and direct cycling connections increase both cycling rates and user satisfaction. Through network analysis, the best locations and methods can be identified to create an effective cycling network that allows cyclists to travel from A to B without unnecessary detours.
By Ane Rahbek Vierø, Anastassia Vybornova, Clément Sebastiao, and Michael Szell. Networks, Data, and Society (NERDS), Computer Science Department, IT Universitet i København
What Is Network-Based Cycling Planning?
Network-based cycling planning focuses not only on the number of bike paths but also on the overall structure and connectivity of the cycling network. A cycling network can consist of bike paths, bike lanes, or any bike-appropriate roads and trails in an area. Common to all of these is that they should be safe and comfortable for all cyclists, depending on local conditions and context (Schoner & Levinson, 2014). See Figure 2 for an example.
Figure 2: A segment of the cycling network in Copenhagen and Frederiksberg.
Why Use Network-Based Cycling Planning?
The first step in analyzing cycling conditions is often to examine the number of bike paths and cycle-friendly routes. More bike paths generally lead to an increase in cyclists and a greater sense of security (Buehler & Dill, 2016; Fosgerau et al., 2023). However, research also shows that the structure of the network significantly impacts cycling rates and cyclist satisfaction (Schön et al., 2024).
A direct and well-connected cycling network is crucial to avoid long and inconvenient detours for cyclists, especially where they may need to avoid high-traffic roads. Fragmented networks with missing links increase both perceived insecurity and the risk of accidents. Unfortunately, cycling networks are often much more fragmented than road networks, with many ‘gaps’ and missing connections.
These deficiencies affect all cyclists but particularly impact children, inexperienced cyclists, the elderly, and cyclists with disabilities. It is therefore essential not only to consider the number of bike paths but also to focus on creating cohesive and direct cycling connections.
How to Apply Network-Based Cycling Planning
The first step in network-oriented cycling planning is to determine the status of the existing network:
- Areas With No Existing Cycling Network:
Planning starts from scratch by choosing a development strategy (Figure 3A) (Szell et al., 2022). - Partially Developed but Fragmented Networks:
The goal is to connect existing paths. Network analysis can identify how to link separate segments into a cohesive network (Figure 3B) (Natera Orozco et al., 2020). - Developed but Incomplete Networks:
The focus is on closing crucial gaps. Network analysis can highlight where new paths will benefit the most cyclists (Figure 3C) (Vybornova et al., 2023). - Existing Strategic Plans:
Use network analysis to prioritize where to begin implementation for the fastest cohesive network development (Figure 3D) (Sebastiao, 2024).
Figure 3: Different stages of cycling networks require different development strategies (Vybornova, Szell, & Sebastiao).
Regardless of how developed a cycling network is, it is important to define clear goals and adapt the network strategy accordingly, as different types of networks serve different cyclists and trip types.
For example:
- Cycle Superhighways: Long, direct routes covering large areas suitable for commuting and long-distance cycling.
- School Routes: Covering smaller areas but requiring many local connections for safety and accessibility.
Building a cycling network can take many years, especially in areas with limited infrastructure. While the long-term goal is a fully developed network, the right development strategy can help create a functional network as quickly as possible.
Examples of Prioritization Strategies:
- Establish paths on the busiest routes first to create broad geographic coverage quickly (Figure 4A).
- Focus on creating a dense, well-connected network with minimal detours (Figure 4B).
Regardless of the chosen planning criteria, targeted strategies generally lead to a cohesive and functional network faster and more efficiently than a random development approach (Szell et al., 2022) (Figure 4C).
Conclusion
Network-based cycling planning supports cohesive and safe cycling connections, promoting a systemic approach to cycling infrastructure development. For more information on network analysis in cycling planning, visit growbike.net, fixbike.net, and linkbike.net, or contact the article’s authors at nerds.itu.dk.
Figure 4: A: Strategy prioritizing central connections. B: Strategy for a dense and well-connected network. C: Random prioritization of new links. Although the final result is the same, there are significant differences in network coherence during development (Szell).
References
Buehler, R. and Dill, J. (2016). Bikeway Networks: A Review of Effects on Cycling. Transport Reviews, 36(1):9–27. Publisher: Routledge _eprint:
https://doi.org/10.1080/01441647.2015.1069908.
Fosgerau, M., Łukawska, M., Paulsen, M., and Rasmussen, T. K. (2023). Bikeability and the induced demand for cycling. Proceedings of the National Academy of Sciences,
120(16):e2220515120. Publisher: Proceedings of the National Academy of Sciences.
Natera Orozco, L. G., Battiston, F., Iñiguez, G., and Szell, M. (2020). Data-driven strategies for optimal bicycle network growth. Royal Society Open Science, 7(12):201130.
Schoner, J. E. and Levinson, D. M. (2014). The missing link: bicycle infrastructure networks and ridership in 74 US cities. Transportation, 41(6):1187–1204.
Schön, P., Heinen, E., Rangul, V., Sund, E. R., and Manum, B. (2024). The impact of street network connectivity on active school travel: Norway’s HUNT study. Environment and Planning
B: Urban Analytics and City Science, page 23998083241235978. Publisher: SAGE Publications Ltd STM.
Sebastiao, C. (2024). csebastiao/orderbike. original-date: 2024-01-11T14:12:43Z.
Szell, M., Mimar, S., Perlman, T., Ghoshal, G., and Sinatra, R. (2022). Growing urban bicycle networks. Scientific Reports, 12(1):6765. Number: 1 Publisher: Nature Publishing Group.
Vybornova, A., Cunha, T., Gühnemann, A., and Szell, M. (2023). Automated Detection of Missing Links in Bicycle Networks. Geographical Analysis, 55(2):239–267.